Stefanos Papanikolaou
Name
Stefanos
Surname
Papanikolaou
Department
NOMATEN
Group
Materials Structure, Informatics and Function
Personal webpage
http://nomaten.ncbj.gov.pl/
Research focus
Materials Informatics, Multiscale Materials Modeling, Statistical Mechanics, alloy composition search and machine learning, Machine learning of strain images, machine learning of interatomic potentials
ML expertise
Unsupervised Machine Learning using Principal Component Analysis and complex clustering,
Supervised Machine Learning using deep convolutional neural networks (Tensorflow), Decision Trees, Support Vector Machines (sklearn)
Relevant publications
- Papanikolaou, S., 2020. Microstructural inelastic fingerprints and data-rich predictions of plasticity and damage in solids. Computational Mechanics, 66(1), pp.141-154. https://link.springer.com/article/10.1007/s00466-020-01845-x
- Yang, Z., Papanikolaou, S., Reid, A.C., Liao, W.K., Choudhary, A.N., Campbell, C. and Agrawal, A., 2020. Learning to predict crystal plasticity at the nanoscale: Deep residual networks and size effects in uniaxial compression discrete dislocation simulations. Scientific reports, 10(1), pp.1-14. https://www.nature.com/articles/s41598-020-65157-z
- Papanikolaou, S. and Tzimas, M., 2019. Effects of rate, size, and prior deformation in microcrystal plasticity. Mechanics and Physics of Solids at Micro‐and Nano‐Scales, pp.25-54.
- Papanikolaou, S., Tzimas, M., Reid, A.C. and Langer, S.A., 2019. Spatial strain correlations, machine learning, and deformation history in crystal plasticity. Physical Review E, 99(5), p.053003. https://journals.aps.org/pre/abstract/10.1103/PhysRevE.99.053003
Other
Enjoying drinking coffee during random hours
Group membership
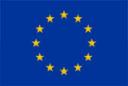
Copyright NOMATEN 2017-2024
