NOMATEN HYBRID-SEMINAR May 22: Machine Learning in Protein Classification, Modeling and Design
NOMATEN HYBRID-SEMINAR
online: https://meet.goto.com/NCBJmeetings/nomaten-seminar
In-person: NOMATEN seminar room (102)
Thursday, MAY 22th 2025 1 PM (CET)
Machine Learning in Protein Classification, Modeling and Design
Dominik Gront, Ph.D., D.Sc.
University of Warsaw
Abstract:
Machine learning has been applied in the natural sciences for many years, including in bioinformatics and molecular modeling. However, it is only within the last decade that the rapid development of deep learning, along with the increased availability of data and computational power, has enabled a qualitative leap in the application of these methods. In my presentation, I will showcase selected examples of machine learning techniques developed and applied in our laboratory, illustrating their potential in protein analysis and modeling.
The first example involves the use of clustering methods to classify cytochrome P450 sequences, employing similarity measures based on local alignments and unsupervised grouping algorithms. The outcome of this work is P450Atlas: an automated tool for detecting and annotating new sequences within the P450 superfamily. The second example demonstrates the application of deep convolutional neural networks to support coarse-grained methods in protein structure modeling. Our method, deepBBQ, enables the reconstruction of complete protein backbone structures based solely on the positions of Cα atoms. The model, trained on a large structural dataset, achieves accuracy comparable to experimental data.
In a third project, we focus on the application of generative deep learning models (e.g., variational autoencoders) to generate realistic conformations of protein fragments. The aim of this approach is to enrich the conformational space in molecular simulations and improve the modeling of intrinsically disordered or partially known protein structures.
During the presentation, I will also discuss the challenges of training machine learning models on biological data and potential directions for further development of these methods in the context of biomolecular modeling.
Bio:
Dominik Gront received his Ph.D. in chemical sciences with distinction in 2006 from the Faculty of Chemistry at the University of Warsaw. He subsequently completed two international postdoctoral fellowships: at the University of Virginia in Charlottesville in the group of Prof. Władek Minor, and at the University of Washington in the laboratory of Prof. David Baker. In 2016, he obtained his habilitation at the Faculty of Chemistry of the University of Warsaw, where he has been employed as an associate professor since 2020. Dr. Gront’s scientific work focuses on the development of generative neural networks for protein modeling and the classification of proteins from the cytochrome P450 superfamily. Since 2019, Dr. Gront has also been a board member of Rosetta Commons, an organization dedicated to the development and maintenance of the Rosetta software suite.
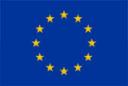
