NOMATEN develops software for better understanding of microscopy images of materials under extreme conditions
NOMATEN develops software for better understanding of microscopy images of materials under extreme conditions
Large Data is common across materials science. Cross-correlating and understanding data from material surfaces is very important since there is typically a direct connection to physical properties. Also, it is very important to use this data to reconstruct the actual microstructure and perform simulations in a computer at the molecular or larger scale. However, understanding of Large Data requires the application of statistical and machine learning techniques. Further, simulations require efficient microstructural reconstruction techniques. Stefanos Papanikolaou's group at NOMATEN investigates such issues.
S. Papanikolaou, Research Group Leader at NOMATEN
The software under development provides solutions that are fine-tuned for efficiently and confluently performing dedicated tasks that allow the understanding and simulation of mechanical behavior. This software will be used by experimenters without any specific knowledge of how and why machine learning works - Stefanos Papanikolaou PhD said. He is a Research Group Leader for Materials Informatics - Structure and Function (MASIF) at NOMATEN.
Microscopy (optical, electron, force, etc.) represents the key approach to "looking" into a material's microstructure and behavior. Microscopy images are typically connected to physical properties of materials and can unveil the type of prior physical processing routes. Materials under extreme conditions, such as high-temperature, large strain and irradiation, undergo microstructural changes that are hard to explain through traditional modeling approaches.
In these cases, AI approaches are key to capturing the changes and connecting them to particular processing routes and physical properties. Currently, the software is open-source. There is a discussion about providing dedicated services to clients that need specific solutions, in similar ways that other popular software is provided - Stefanos Papanikolaou comments.
The origin of the idea is coming from Papanikolaou's development of prior software on key dimensional reduction techniques and the application of convolutional neural networks machine learning (SeaPy software). The premise has been that the machine learning software landscape is currently at the same position as traditional software in the dotcom era (1995-2000): A lot of solutions exist, but only experts know how to apply them and acquire meaningful results. Currently, there is a worldwide need for dedicated machine learning solutions to specific applications, that experimenters and practitioners can readily apply to their library datasets and get meaningful results. The Papanikolaou's group is working towards dedicated solutions for microstructural and mechanical applications.
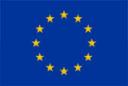
